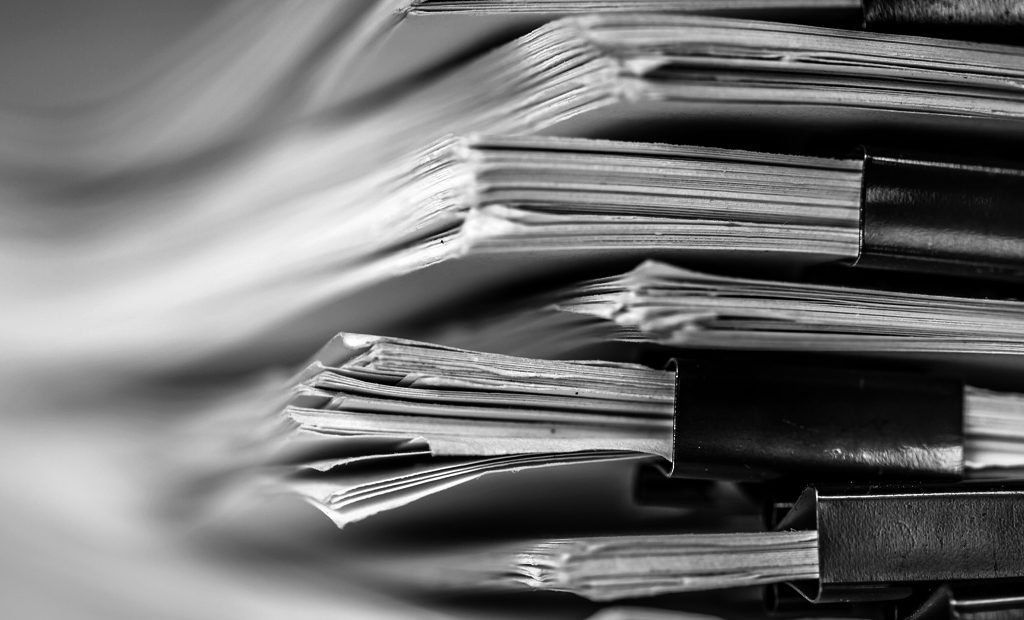
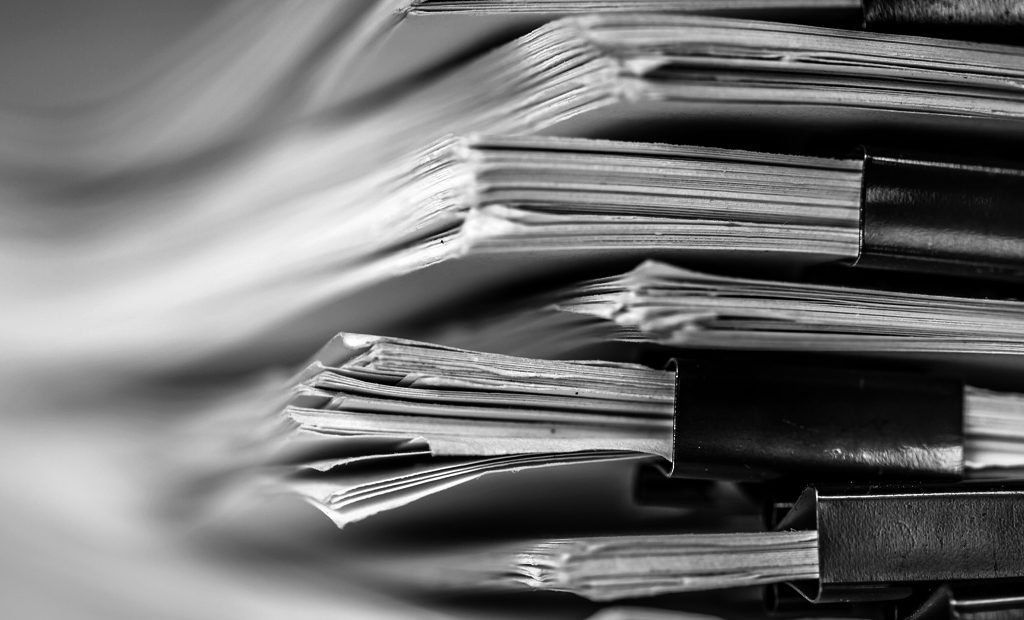
How to Build a Strong Scientific Claim to Satisfy Skeptical Minds
When a research group submits a scientific paper for publication in a journal, they bite their nails and chatter their teeth while the paper undergoes review by three of their peers. When that fateful email comes in with their feedback, it usually looks something like this:
- Reviewer 1 requires a particular set of revisions to the paper
- Reviewer 2 requires completely contradictory revisions
- Reviewer 3 tells you that you must start over
Researchers often face this for the first time as graduate students. After they overcome the initial shock, these students learn how to scrutinize their own work and design experiments that will pass muster among their peers in the scientific community. By extension, this experience teaches scientific researchers how to question others’ scientific claims.
We find the same skepticism in the scientific trade media. Journalists who focus on science and healthcare receive dozens of pitches a day, with some needing revisions. Some pitches scream “compensation” by trying to oversell a company using buzzwords, while others showcase due diligence to ensure the presentation of a strong story with data to back it up. Science and healthcare journalists train themselves to tell the difference because their audience depends on it.
If a company is targeting scientists or healthcare professionals (HCPs) through the scientific trade media, it needs to make a strong scientific claim that these audiences will accept. Therefore, when pitching the media, it helps to have scientists and HCPs on hand who know how to design experiments and scrutinize scientific data. These individuals know how to ensure a story meets the standards of the scientific community from which a company is seeking credibility.
Here, we describe what these audiences are looking for through our experience as scientists and HCPs. We explain how we would design a scientific study with results people can trust.


The Randomized Controlled Trial
The current gold standard experimental design for drug research in humans is the randomized controlled trial (RCT). RCTs, including double-blind RCTs, became the go-to design not only because they improve safety for research participants and the public, but also because they give researchers the best hope of revealing the true effects of an experimental drug.
While most people only hear the terms “double-blind,” “randomized,” and “controlled” in the context of clinical trials, these terms are actually critical elements of most legitimate experimental designs, whether they involve humans, animals, cells, or liquid samples. Incorporating all three of these elements into an experimental design goes a long way in establishing the credibility of a study. So, what do these terms mean?
Double-Blind
In a double-blind experiment, neither the researcher nor the study participant knows which treatment they are receiving. This design helps preserve the integrity of a study in several ways. First, if a researcher or participant knows which treatment is being administered, he or she may skew the experimental conditions or data to generate a result they want to see.
For example, a researcher may nudge participants or the data itself toward a direction that confirms their hypothesis. Sometimes this is intentional, but more often, this bias (called researcher bias or experimenter bias) is subconscious. On the flip side, if a participant knows they are receiving the study treatment, they may experience the placebo effect. This is when the body or mind responds to treatment, whether it works or not—just because the person expects the treatment to improve their health.
Randomized
It’s said that according to the data, everyone has the personality, cognitive abilities, and memory of an undergraduate psychology student. This is because most undergraduate psychology courses offer credit to students for participating in psychology studies, and as a result, the study populations for these studies tend to all look the same. But obviously, this population does not necessarily reflect the broader public. Randomization aims to fix this problem.
In an experiment, randomization means arbitrarily deciding which subjects in a broader population get studied and which treatment each one receives. In an ideal setting, researchers will pick a random group of humans, animals, or cells from the broader population (i.e. a random sample) to run their experiment on. This way, researchers can ensure that the pool of research subjects accurately represents the broader population (not just undergraduates).
Within this subject pool, researchers will randomly decide which individual subjects receive each treatment. Here, their goal is to make sure all participating groups are about equal in all the ways that matter. This way, researchers will be able to ascribe any differences they see between groups to the different treatment conditions alone, and not to some other difference between them.
Researchers will often confirm the similarity between their groups by testing them on several baseline measures and reporting these data in their papers. If the groups differ significantly before receiving treatment, their peers start to question the validity of the results.
Controlled
If you give a sick person a drug and they improve, can you attribute their improvement to the drug? Not necessarily. It is possible this person improved for some reason that had nothing to do with the treatment, including something as simple as the passage of time.
To know whether they can attribute the change in their research subject to the treatment, researchers run controls. These are often in the form of a placebo or a sham (pretend) treatment. Researchers aim to create a placebo experience that is as similar to the real thing as possible. For instance, if the treatment is an infusion, the placebo group receives an infusion of saline. If the treatment in an animal study is a surgery, the sham group will undergo all the same prep work, cuts, and recovery as the treatment group, but the actual surgical intervention will not happen. Similarly, cells in a control group face the same environmental conditions as the test group but do not receive the treatment under question.
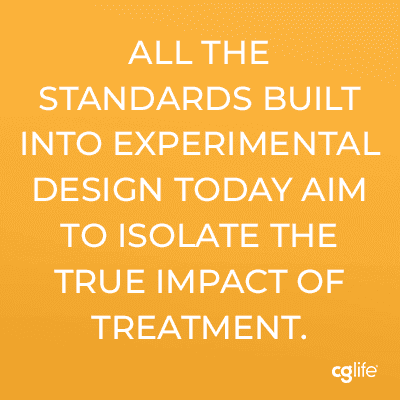
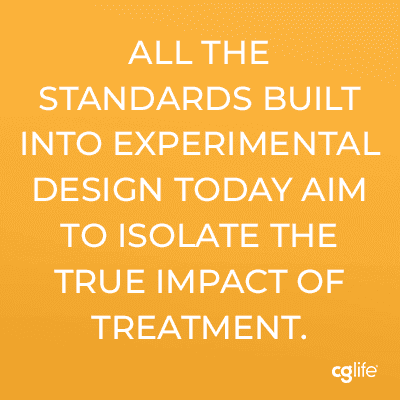
Researchers create such complex placebos for two reasons: a) to keep the study blind to human participants and b) to create a similar experience for all the research subjects to reduce the number of differences between them. Generally, data from the placebo group will tell researchers if and how people change in the absence of receiving the true treatment, which helps them isolate the effect of the treatment itself.
Some experiments use complex molecular tests to assess the impact of a treatment. Researchers cannot always trust that their test went to plan, so they will run positive controls to make sure. These controls are treatments with a known, reliable effect. If the test picks up the expected effect of the positive control treatment, researchers can be reasonably certain that their test works and that their experimental protocol will capture the true effect of the experimental treatment.
A poorly controlled experiment once led researchers to believe they discovered a certain cancer marker, EPOR, that resided on tumor cells. Millions of dollars and a failed clinical trial later, they found that their cancer marker was not actually present on tumor cells and that their test initially identified the wrong protein as EPOR. In fact, instead of EPOR, they detected a protein that is present in all of our cells and has no role in cancer.
Additional Safeguards
All the standards built into experimental design today aim to isolate the true impact of treatment. This helps researchers minimize ambiguity in their experimental results. In addition to these safeguards, researchers also make sure their experimental population is large enough. If the population is too small, researchers may not detect a subtle treatment effect. Conversely, if researchers detect a significant effect in a small research population, it is hard to determine if the findings represent how a larger population would respond.
Another sign of a strong experiment in humans is the use of quantitative measurements. Instead of having participants estimate their health or behavior, such as their alcoholic drink consumption or pain level over the past month, researchers aim to make their measurements more objective. For example, in a study of diet, rather than asking someone to estimate their calorie intake over a week, a researcher might ask them to keep a journal of all the food they ate. This, again, prevents bias and increases the credibility of the data.


Finally, when scrutinizing a scientific study, scientists often seek information on whether the researchers have any conflicts of interest (COIs) and whether these COIs were properly disclosed. Researchers can be biased to demonstrate a result based on the financial incentives they receive. While these COIs are not necessarily bad, it’s important for researchers to be honest about them so their data can be interpreted within the full context of their research and professional activity.
Why This is Important
Companies seeking credibility among the scientific community need to ensure their science is sound. Scientists, HCPs, and science journalists are trained to scrutinize scientific claims and they can tell the difference between a sales pitch and true, impactful scientific results.
Sure, you can use our team of Ph.D. scientists and HCPs to translate your science into engaging content. But you can also use us as a focus group to determine if your science is up to snuff and as advisors to help you build a strong scientific story for your audience. If you want help testing and demonstrating the credibility of your scientific story, contact us here.